The Perils and Promise of Automated Content Analysis in Non-English Languages
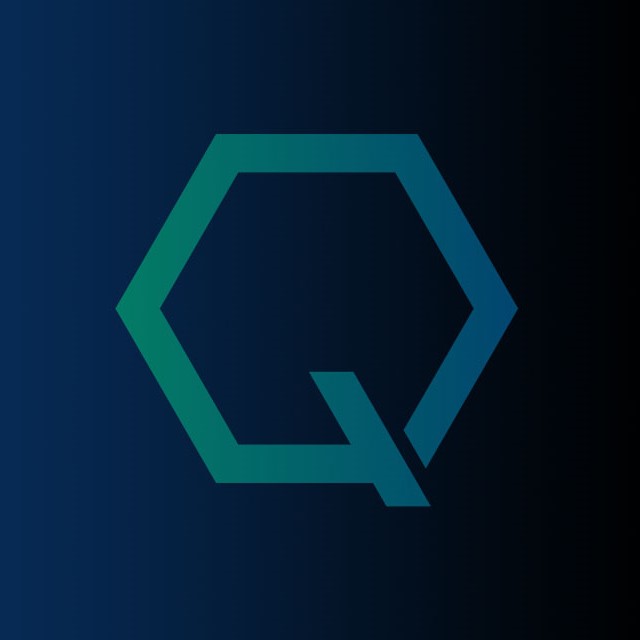
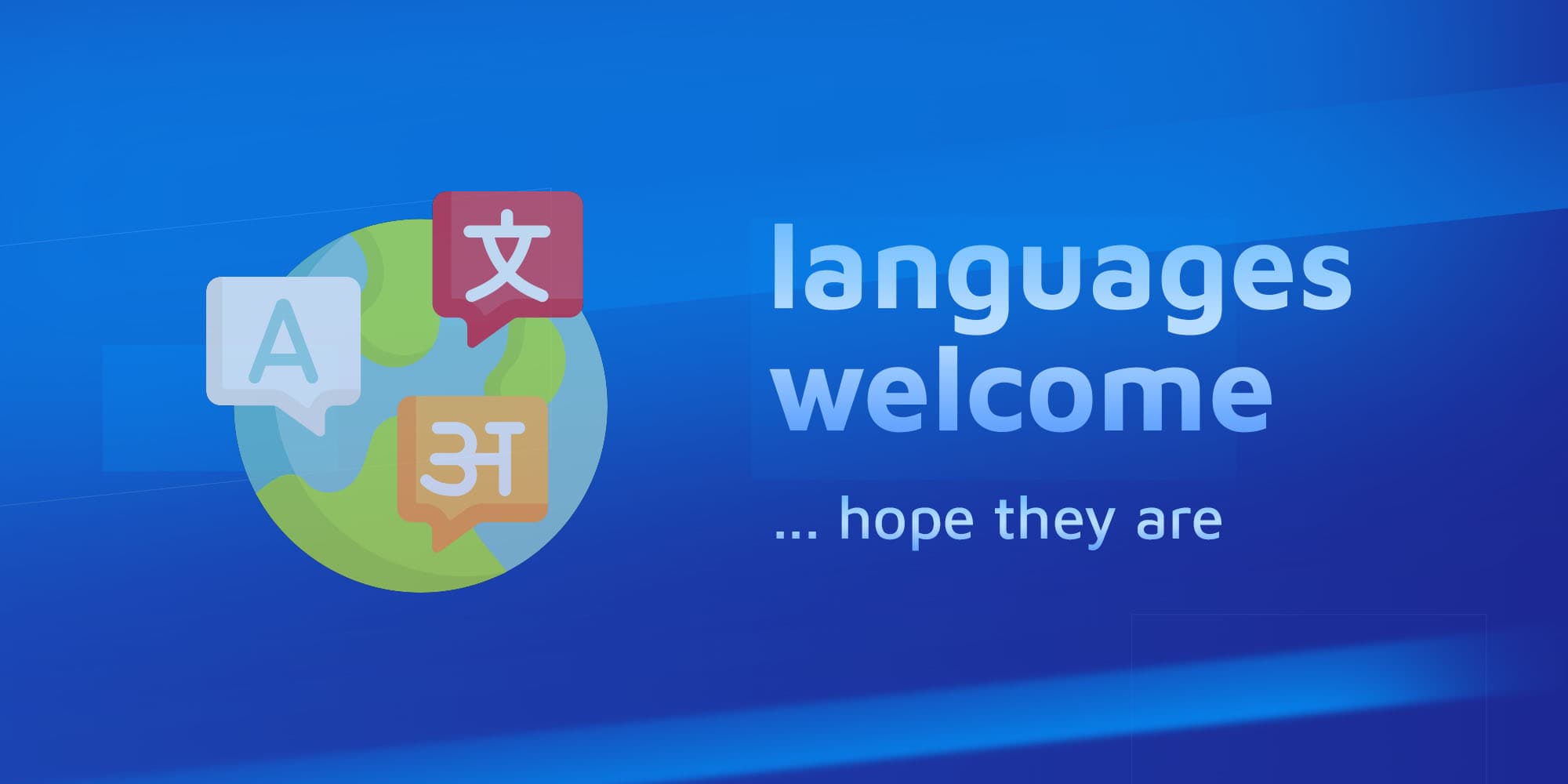
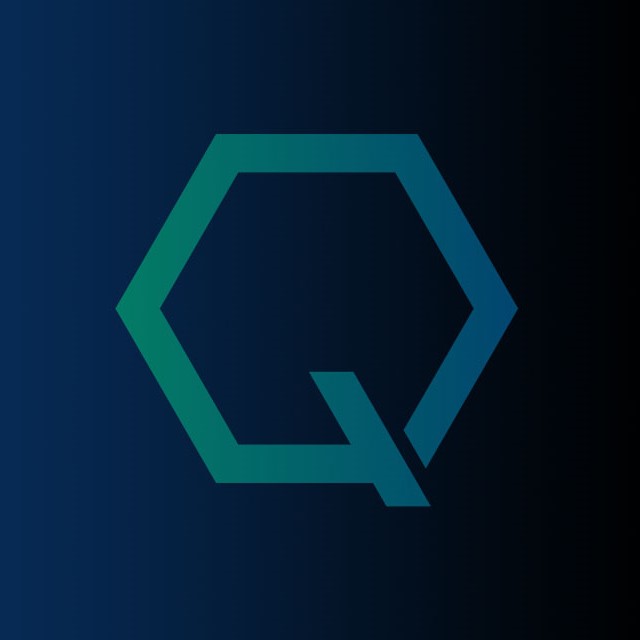
Automated content analysis systems have become ubiquitous - we encounter them when applying for jobs, interacting with customer service, searching for information online, or appealing content moderation decisions on social media.
While these systems can be useful, they also have severe limitations, often failing to account for the context and nuance of language. These problems are amplified when dealing with languages other than English.
Vast Differences in Data and Research
The availability of training data and specialized software tools varies tremendously between languages. English is by far the most "resourced" language, with orders of magnitude more digitized data available compared to any other language. English is also the overwhelming focus of natural language processing (NLP) research.
According to the Center for Democracy and Technology, while languages like Arabic, German, Mandarin, Japanese and Spanish have millions of data points available, they are still researched and commercialized much less than English.
Many other widely spoken languages like Bengali and Indonesian have far less data, and most Indigenous and endangered languages have little to none. Even within high-resource languages, there are often "data voids" for variants like African-American Vernacular English, dialects like Hindi/Urdu, and multilingual speech like Spanglish.
Risks of Failure and Harm
The lack of data, tools and research for non-English languages has led to content analysis failures with major real-world consequences, without naming names.
Given the known struggles of even English content analysis, automated systems are almost certainly failing in damaging ways when used in non-English contexts like immigration proceedings or predictive policing. Deploying faulty translation and analysis tools, especially for high-stakes decisions about blocking content, denying benefits or reporting people to authorities, poses significant risks.
Efforts to Bridge the Language Gap
In recent years, there have been increasing efforts to close the computational divide between English and other languages. This sometimes involves building new tools and datasets for specific under-resourced languages.
One example is Uli, a browser plug-in to detect hate speech and online abuse in Hindi, Tamil and Indian English. To create Uli, two India-based NGOs recruited diverse volunteers and experts to first define the boundaries of this type of speech, and then annotate tweets to develop custom datasets in each language.
In other cases, instead of collecting more data, researchers find ways to stretch limited data further using large language models. These models, trained on billions of mostly English words, have set performance records in many tasks including machine translation with only minor tweaking for new languages.
However, their robustness and interpretability are questionable, so using them for non-English content analysis may be pushing their capabilities to or past the limit.
This is where Lexiqn is attempting to play a part in. As everyone else these days, it does leverage large language models for research but first, and more importantly, it starts by providing a workflow for seamless ingestion, translation and analysis of content from under-resourced languages.
It puts together a set of diverse tools: from optical character recognition (OCR) and translation models during ingestion, to unique, in-house developed, data analysis, cataloging and indexing algorithms within its database. The database process is the brains behind Lexiqn constantly trying to understand the content more as the user interacts with it.
The above is done before content is eventually provided to the large language models for the ubiquitous generative purposes we are enjoying today.
The work is challenging and requires lots of granular adjustments due to the complex nuances within languages but we believe it is rewarding. If not immediately, at least, we are hopeful someone will be inspired to come up with an even better solution.
The Path Forward
As automated content analysis systems continue to proliferate, the risks they pose, particularly for speakers of low-resource languages, must be addressed properly. To improve these systems and protect against potential harm, we first need to understand their current limitations.
The goal is to make this complex issue accessible to policymakers, civil society, journalists and the public to inform efforts to study and mitigate the risks. Only by shining a light on how these systems work can we hope to make them work better for everyone.
But on the technical side, as engineers, we should strive to provide the tooling for the aforementioned entities and personalities to interact with content that is so rare or foreign and yet so rich, that they would think they were missing out.